Download the above schedule as a .pdf.
Following is a list of confirmed speakers. Apart from these, 20 German and 10 Indian students would make presentations of 15 minutes each.
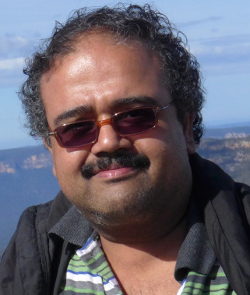 | Ravindran Balaraman [Biography]
Ravi is an associate professor at the Department of Computer Science and Engineering at the Indian Institute of Technology Madras. He completed Ph.D. at the Department of Computer Science, University of Massachusetts, Amherst. He worked with Prof. Andrew G. Barto on an algebraic framework for abstraction in Reinforcement Learning.
His current research interests span the broader area of machine learning, ranging from Spatio-temporal Abstractions in Reinforcement Learning to social network analysis and Data/Text Mining. Much of the work in his group is directed toward understanding interactions and learning from them.
IIT Madras, India
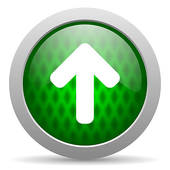
|
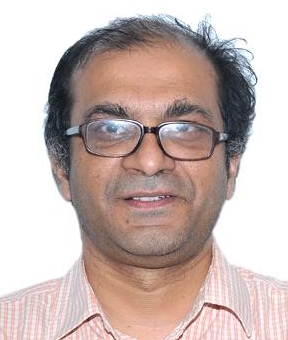 | Chiranjib Bhattacharyya [Biography]
IISc Bangalore, India
Learning Probabilistic Topic models -- A SVD based approach
Discovering topics from large text corpora is an important problem in Text
Analytics. Recently there has been interest in designing algorithms
which can provably recover Topics from a finite number of samples. It is
widely believed that Singular Value Decomposition(SVD) based Algorithms
cannot guarantee recovery of topics.
In this talk we will discuss some recent results which shows that SVD
based algorithms can recover Topics if the data
satisfies some assumptions. We will present empirical evidence in support
of our assumption and also show that
the proposed SVD based procedure outperforms the state of the art.
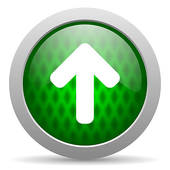
|
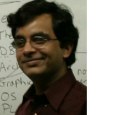 | Venkatesan Chakaravarthy [Biography]
Venkatesan Chakaravarthy is a member of the high performance computing group
at IBM Research - India. Broadly, he is interested in theoretical computer
science and its applications to areas such as database systems and high
performance computing. His current research focuses on approximation
algorithms for scheduling problems, and developing scalable
algorithms and implementations for graph theoretic problems on
distributed platforms such as IBM BlueGene systems.
IBM Research, India
Scalable Graph Analytics: Single Source Shortest Path and Subgraph Couting
We shall discuss two graph theoretic problems, single source shortest path and
subgraph counting, from the perspective of developing high performance
algorithms for large distributed memory systems.
In the single-source shortest path problem,
the goal is to find the shortest paths from a source vertex
to all other vertices in a given edge weighted graph.
We describe a parallel algorithm, derived from the Bellman-Ford and $\Delta$-stepping
algorithms. The algorithm employs various pruning techniques such
as edge classification and direction-optimization to reduce inter-node communication traffic.
Furthermore, load balancing strategies are used to handle higher-degree vertices.
In the largest tested configuration, a synthetic R-MAT graph with 250 billion vertices and
four trillion edges on a BlueGene/Q system with half a million cores,
the implementation achieved a processing rate of three Trillion Edges Per Second (TTEPS),
a four orders of magnitude improvement over the best published results.
In the subgraph counting problem, the goal is to count the number of
occurrences of a small query (say, less than 10 nodes) in a large data graph
(millions of vertices). Many important special cases (e.g. triangles, trees,
$4$- and $5$- node queries) have received significant attention.
Color coding has been proposed as a general and powerful algorithmic technique
for subgraph counting. The technique has been shown to be effective in several
applications, but scalable distributed implementations are only known for
the special case of tree queries (i.e. queries of treewidth one).
We describe the first efficient distributed implementation
for color coding that goes beyond tree queries: our
algorithm applies to any query graph of treewidth 2.
The algorithm structures the computation to work around
high degree vertices in the data graph, and achieves good
runtime and scalability on a diverse collection of data and query graph pairs.
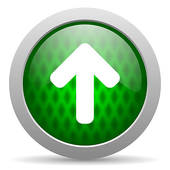
|
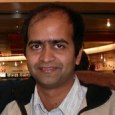 | Prasad Deshpande [Biography]
Prasad M. Deshpande is the CTO and Co-founder of Iqlect, a startup in the area of real time analytics. Prior to this, he was a Senior Technical Staff Member and Manager at IBM Research - India. His areas of expertise lie in data management, specifically data integration, OLAP, data mining and text analytics. He received a B. Tech in Computer Science and Engineering from IIT, Bombay and M.S. and Ph.D. degrees in Database Systems from the University of Wisconsin, Madison. He is an ACM Distinguished Scientist and was a member of the IBM Academy of Technology. His current focus is in the areas of big data and real time analytics. He has more than 40 publications in reputed conferences and journals and 14 patents issued. He has served on the Program Committees of many conferences, as a PC member and PC Co-Chair.
IBM Research Bangalore, India
Discovering Big Data
Data discovery deals with the problem of discovering useful data from a proliferation of different types and sources of data. Businesses are looking for rapidly available, differentiating insights gleaned from sources such as proprietary databases, social media, and commercially and publicly available datasets. However, a major block to using such datasets is that the schema and semantics of these datasets is not known beforehand. Further, the data could be various types such as structured, unstructured or semi-structured. The exact analysis to be run on the data is also not known beforehand. There is a need to support ad-hoc self-service analytics on such large collections of data. To achieve this, it is necessary to catalog, curate and govern these assets so they can be found, understood and reused by the enterprise. In this talk, I will describe the BRIDGE data discovery system that we built at IBM to automatically discover rich relationships between different data assets and enriches the metadata so that the right datasets can be found when required. Further, I will also touch upon some real time scenarios where we may need to discover changing characteristics of streaming data.
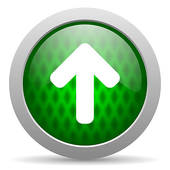
|
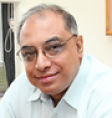 | Sumit Ganguly [Biography]
Sumit Ganguly completed his B.Tech from IIT Kanpur in 1987 and MS and PhD from the University of Texas at Austin in 1992. He was an Assistant Professor in Computer Science at Rutgers University for a few years before joining IIT Kanpur in 1997. He has worked in parallel and deductive database systems, query optimization and now works in data stream algorithms.
IIT Kanpur, India
Estimating High Frequency Moments of Data Streams
In the data streaming model, data in the form of a large (and possibly interminable) sequence of updates of the form (i,v) arrive at a server, where, i denotes an item from a domain of possible items (IP addresses, pairs of IPs, etc.) which is abstracted as {1,2, …, n} and v is the change in the frequency of i. The frequency is an n-dimensional vector f whose ith coordinate at an instant is the sum of the changes to the frequency of i made by the updates that have arrived till this instant. This model is known as the turnstile model for data streaming. In this model, an algorithm may use sub-linear memory to store a summary of the data. The data is also processed as it arrives, i.e., in an online fashion. A query on the data stream may be answered by only using the summary data stored.
This model is useful for rapid prediction and monitoring applications. An influential problem in this area is the estimation of the p th frequency moment F_p defined as the pth power of the absolute value of the frequencies (i.e., \norm{f}_p^p). The estimation problem is to compute an estimate \hat{F}_p for F-p that is within a multiplicative factor of (1 \pm \epsilon) of Fp with confidence 7/8, where, \epsilon is a given parameter. The problem has been studied substantially in the literature. We present a summary of the developments and present a recent algorithm.
For $0 < \epsilon \le 1$, the algorithm uses space $O( n^{1-2/p} \epsilon^{-2} +
n^{1-2/p} \epsilon^{-4/p} \log (n))$ words. This improves over the current bound of $O(n^{1-2/p} \epsilon^{-2-4/p} \log (n))$ words by Andoni et. al. in \cite{ako:arxiv10}. Our space upper bound matches the lower bound of Li and Woodruff \cite{liwood:random13} for $\epsilon = (\log (n))^{-\Omega(1)}$ and the lowerbound of Andoni et. al. \cite{anpw:icalp13} for $\epsilon = \Omega(1)$.
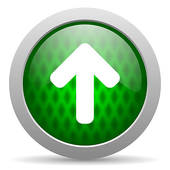
|
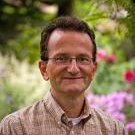 | George Karypis [Biography]
George Karypis is an ADC Chair of Digital Technology Professor at the Department of Computer Science & Engineering at the University of Minnesota, Twin Cities. His research interests spans the areas of data mining, high performance computing, information retrieval, collaborative filtering, bioinformatics, cheminformatics, and scientific computing. His research has resulted in the development of software libraries for serial and parallel graph partitioning (METIS and ParMETIS), hypergraph partitioning (hMETIS), for parallel Cholesky factorization (PSPASES), for collaborative filtering-based recommendation algorithms (SUGGEST), clustering high dimensional datasets (CLUTO), finding frequent patterns in diverse datasets (PAFI), and for protein secondary structure prediction (YASSPP). He has coauthored over 250 papers on these topics and two books (“Introduction to Protein Structure Prediction: Methods and Algorithms” (Wiley, 2010) and “Introduction to Parallel Computing” (Publ. Addison Wesley, 2003, 2nd edition)). In addition, he is serving on the program committees of many conferences and workshops on these topics, and on the editorial boards of the IEEE Transactions on Knowledge and Data Engineering, ACM Transactions on Knowledge Discovery from Data, Data Mining and Knowledge Discovery, Social Network Analysis and Data Mining Journal, International Journal of Data Mining and Bioinformatics, the journal on Current Proteomics, Advances in Bioinformatics, and Biomedicine and Biotechnology.
University of Minnesota, USA
Recent Advances in Recommender Systems and Future Directions
Recommender systems are designed to identify the items that a user will like or find useful based on the user’s prior preferences and activities. These systems have become ubiquitous and are an essential tool for information filtering and (e-)commerce. Over the years, collaborative filtering, which derive these recommendations by leveraging past activities of groups of users, has emerged as the most prominent approach for solving this problem. Among the multitude of methods that have been developed, user- and item-based nearest-neighbor approaches are the simplest to understand and are easily extended to capture different user behavioral models and types of available information. However, in their classical forms, the performance of these methods is worse than latent-space approaches.
This talk presents an overview of recent methodological advances in developing nearest-neighbor-based recommender systems that have substantially improved their performance. The key components in these methods are: (i) the use of statistical learning to estimate from the data the desired user-user and item-item similarity matrices, (ii) the use of lower-dimensional representations to handle issues associated with sparsity, (iii) the combination of neighborhood and latent space models, and (iv) the direct incorporation of auxiliary information during model estimation. The talk will provide illustrative examples for these methods in the context of item-item nearest-neighbor methods for rating prediction and Top-N recommendation. In addition, the talk will present an overview of exciting new application areas of recommender systems along with the challenges and opportunities associated with them.
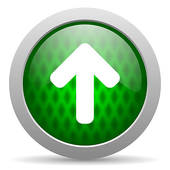
|
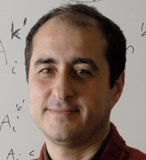 | Stefano Leonardi [Biography]
Stefano Leonardi is Full Professor of Computer Science at Sapienza University of Rome. His research focuses on online and approximation algorithms, web algorithmics and data mining, economics and computation. He is a Senior Research Fellow, Sapienza School for Advanced Studies, and won a Google Focused Award 2014 on Algorithms for large-scale data analysis. He serves on the editorial board the ACM Transactions on Algorithms.
Sapienza University of Rome, Italy
Robust clustering and ranking for large-scale datasets
This talk will present theoretical and experimental work on algorithms for robust clustering and ranking for large-scale datasets. The first part of the talk will present algorithms for universal hierarchical clustering that are robust against outliers. The second part of the talk will present similarity ranking methods that can be efficiently composed at query time.
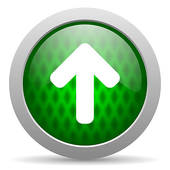
|
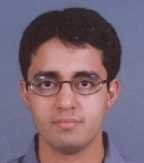 | Sachin Lodha [Biography]
Sachin Lodha is part of TCS’s Corporate Technology Office and works out TCS Innovation Labs - TRDDC, Pune. He has led and shaped TCS’s Data Privacy R&D Program right from its inception in early 2005.
Sachin Lodha graduated with a B.Tech. in Computer Science and Engineering from the Indian Institute of Technology, Bombay, in 1996 and received his Ph.D. in Computer Science from Rutgers University, USA, in 2002. Currently he is a member of TCS’s Corporate Technology Council, and also an ACM India Eminent Speaker.
TCS Innovation Labs Pune, India
How to De-Identify Big Data
In today's hyper networked world with global data flows, almost all major business and technology trends, then be it Big Data, Cloud Computing, Mobility, BYOD, or Internet of Things, require deep privacy underpinnings. It has become increasingly apparent that unless people’s privacy requirements are holistically satisfied, neither the existing businesses would be able to sustain nor new ventures would take off.
In this talk we begin by observing that security and privacy are fundamentally different notions. While security paradigm assumes a clear separation between users and attackers, in privacy paradigm users are attackers too, depending on the use intent, and therefore security controls such as standard encryption based mechanisms do not suffice!
Further we discuss the major goal of data privacy research, that is, to find the right balance between the two extremes of fully disclosed and completely withheld data that preserves both data privacy and its utility, and thus enable data sharing.
We then deliberate on two major privacy frameworks, namely, differential privacy and k-anonymity. We find that the k-anonymity framework is more suitable for several big data contexts that we consider, but it requires us to make the right choice for k, to accurately identify quasi-identifiers, and to design efficient algorithms for making the given dataset k-anonymous with minimal impact on data utility for the given application context. We will present some key results for the same.
Finally we close the talk with a brief overview of TCS Data Privacy Suite that provides efficient and scalable technology for achieving big data privacy.
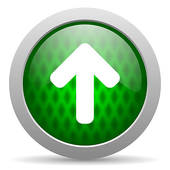
|
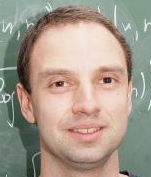 | Ulrich Meyer [Biography]
Ulrich Meyer is a full professor of Algorithm Engineering at Goethe University Frankfurt. He obtained his Ph.D. in Computer Science from the Saarland University Saarbrücken in 2002. Before joining Goethe University in 2007 he was a Postdoc, Research Associate, and senior researcher (at the level of Associate Professor) at Max-Planck Institute for Computer Science. He has also been a Visiting Assistant Professor at Duke University and a Guest Researcher at the Hungarian Academy of Sciences.
His main research area is algorithms for advanced models of computation (e.g. parallel computing and memory hierarchies) with a focus on graph algorithms.
He is currently coordinating the German Research Cluster on Algorithms for big data, funded by the German Research Foundation (DFG).
Goethe University Frankfurt am Main, Germany
Algorithm Engineering for large data sets – some examples.
Basic algorithmic research traditionally assumed some variant of the “von Neumann” model of computation with a single processor and uniform memory. However, more advanced models are by now
an important established part of algorithmic research because many of
the challenges in modern computer science have to do with communication, parallel computing, and memory hierarchies. Especially
in processing large data sets neglecting machine architecture easily becomes a major performance-killer.
In the talk I will review some of our research on graph-algorithms for data residing on secondary memory, and energy-efficient sorting using solid-state disks.
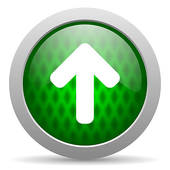
|
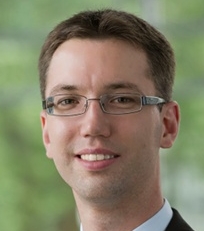 | Henning Meyerhenke [Biography]
Henning Meyerhenke is an Assistant Professor (Juniorprofessor) at the Institute of Theoretical Informatics at Karlsruhe Institute of Technology, Germany, since October 2011. From October 2010 to September 2011 Henning was a postdoctoral researcher in Georgia Tech's College of Computing. Henning received his Diplom degree in Computer Science from Friedrich-Schiller-University Jena, Germany, in 2004 and his Ph.D. (with highest distinction) in Computer Science from the University of Paderborn, Germany, in 2008. After his graduation he was a Research Scientist at NEC Laboratories Europe in Sankt Augustin, Germany, and a Postdoctoral Researcher at the University of Paderborn until September 2010.
His main research interests are in parallel algorithm engineering for massive data sets in three main application areas: Combinatorial scientific computing (graph partitioning, load balancing, multilevel methods), network analysis (community detection, network metrics in dynamic scenarios, NetworKit library), and in algorithmic problems with connection to the life sciences (such as sequence assembly). Recently Henning has acquired significant funding by DFG, BMBF, and MWK Baden-Wuerttemberg. Together with his co-authors, Henning received the Best Algorithms Paper Award at the 22nd IEEE International Parallel and Distributed Processing Symposium (IPDPS'08).
Karlsruher Institut für Technologie, Germany
Scalable algorithms for analyzing large networks with NetworKit
Complex networks are equally attractive and challenging targets for data mining. Novel algorithmic solutions, including parallelization, are required to handle data sets containing billions of connections. We present NetworKit, an open-source software toolkit for efficient graph algorithms and the analysis of such massive complex networks. NetworKit is a hybrid combining the performance of kernels written in C++ with a convenient Python frontend. The package targets shared-memory platforms with OpenMP support.
A few algorithm classes from NetworKit will be presented in some detail, in particular algorithms for community detection and centrality computations. In comparison with related software, we propose NetworKit as a package geared towards large networks and satisfying three important criteria: High performance, interactive workflows, and integration into an ecosystem of tested tools for data analysis and scientific computation.
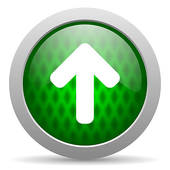
|
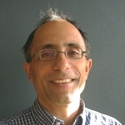 | Rajeev Raman [Biography]
After defending my PhD thesis in October 1991, I took up a Postdoctoral Fellowship in the Algorithms and Complexity Group at the Max-Planck-Institut für Informatik, which is headed by Kurt Mehlhorn. In January 1993 I joined the University of Maryland Institute for Advanced Computer Studies, as a Research Associate working with Uzi Vishkin. Crossing the Atlantic yet again, I joined the Algorithm Design Group at King's College London in 1994. I have been at Leicester since January 2001 and was the Head of the Computer Science Department from 2003 through 2006.
I've been a visiting researcher at the Max-Planck-Institut, Courant Institute, Rutgers, HKUST and Kyoto University.
University of Leicester, UK
Succinct Data Structures for Data Mining
We often use "Big Data" techniques when most users only have "big data". "big data" can often be handled efficiently by applying standard algorithms developed, tried and tested but coupled with succinct data structures to reduce the memory usage of such algorithms, thus allowing the "big data" to be processed in memory. I will introduce succinct data structures and some recent applications to "big data".
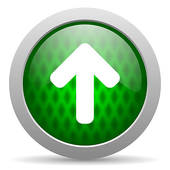
|
 | Sayan Ranu [Biography]
Sayan Ranu is an Assistant Professor at IIT Madras since January, 2014. His research interests include spatio-temporal data analytics, graph indexing and mining, and bioinformatics. Prior to joining IIT Madras, he was a researcher at IBM Research. He obtained his PhD from University of California, Santa Barbara (UCSB) in March 2012. He was a recipient of the "Distinguished Graduate Research Fellowship" at UCSB. He obtained his Bachelor of Science from Iowa State University, where he received the “President’s top 2% of the class” award.
IIT Madras
Mining Communication Motifs from Dynamic Networks
A fundamental problem in behavioral analysis of human interactions
is to understand how communications unfold. In this talk,
we will analyze this problem by mining Communication motifs from dynamic
interaction networks. A communication motif is a recurring
subgraph that has a similar sequence of information flow. Mining
communication motifs requires us to explore the exponential
subgraph search space where existing techniques fail to scale. To
tackle this scalability bottleneck, we will discuss a technique called
COMMIT. COMMIT converts a dynamic graph into a database of
sequences. Through careful analysis in the sequence space, only a
small portion of the exponential search space is accessed to identify
regions embedding communication motifs. Extensive experiments
on three different social networks show COMMIT to be up to two
orders of magnitude faster than baseline techniques. Furthermore,
qualitative analysis demonstrate communication motifs to be effective
in characterizing the recurring patterns of interactions while
also revealing the role that the underlying social network plays in
shaping human behavior.
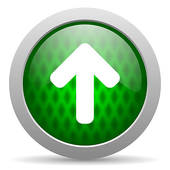
|
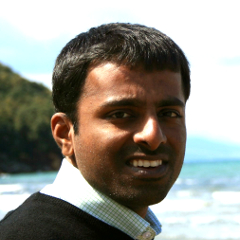 | Uday Reddy [Biography]
Uday Reddy is an Assistant Professor in the Department of Computer
Science and Automation at the Indian Institute of Science, Bangalore, India. His research interests are in the development of programming and compiler/runtime technologies for multicore architectures with an emphasis on high performance and automatic parallelization, the design of domain-specific compilers, and the polyhedral compiler framework. He recently received a Google Research Award (2015) for ongoing research and development on PolyMage. He has also received several other grants and awards for his research, including ones from Intel Labs, National Instruments R&D, AMD, and C-DAC, an NVIDIA CUDA research center award, and an INRIA Associate Team award. Before joining IISc, he was with the Advanced Compiler Technologies group at the IBM T.J. Watson Research Center, Yorktown Heights, New York. He received his PhD in Computer Science and Engineering from the Ohio State University in 2008, and his B-Tech in Computer Science and Engineering from the Indian Institute of Technology, Madras in 2004.
IISc Bangalore, India
Programming and Compiler Technologies for Big Data: efficiently translating algorithms into high-performance code
This talk will present challenges involved in translating big data algorithms into high-performance code in a manner that is also productive for programmers. Big data has a strong connection with high-performance computing due to the need to exploit parallelism and locality when dealing with large amounts of data. We will first look at the relative strengths and weaknesses of various programming approaches: ultra high-level languages, domain-specific languages and code generators, tuned library-based, and completely manual.
An attractive solution that simultaneously provides high programmer productivity and high parallel performance is the design of new domain-specific languages (DSL) and their compilers. I will present our experience with the application domain of image processing pipelines as a motivating example, and emphasize the need for such solutions for emerging computation domains of interest to big data such as deep learning. A short video demo will also be presented.
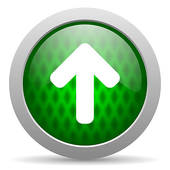
|
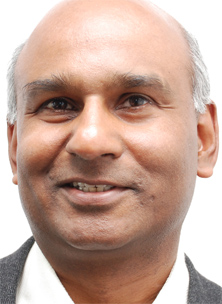 | Anand Srivastav [Biography]
Anand Srivastav studied Mathematics and Physics at the University of
Muenster, Germany from 1978 -- 1984. He completed the PhD in Mathematics
with a thesis in Functional Analysis at the University of Muenster in Spring 1988.
Thereafter, he moved joined the Research Institute of Discrete Mathematics, Uiversity of Bonn,
from 1988 -- 1993. After academic stays as a visitig faculty at the University of
Minnesota, Yale University and New York University, he joined the Free University
of Berlin in 1994, and in 1995 the Humboldt University of Berlin. In Berlin he completed his
Habilitation in Computer Science with a thesis on
,, Derandomzied Algorithms in Combinatorial Optimziation´ in 1996. Since 1997 he
is professor and chair for Discrete Optimization at Kiel University.
From 2000 -- 2005 he has been the speaker
of the DFG research training group 357 (,,Graduiertekolleg´) ,,Efficient Algorithms and
Multiscale Methods´'at Kiel University. Since 2007 he is PI in the DFG cluster of excellence
,,The Future Ocean´ and since 2010 he is speaker of the research platforms of the cluster.
In 2013 he held the guest professorship at the Indo-Max-Planck Center for Computer Science
at IIT Delhi.
His research interests
are combinatorial optimization, discrepancy theory, randomized and derandomized algorithms,
algorithm engineering and big data applications in marine science and life science, with
a personal emphasis on the Indo-German cooperation in computer science and mathematics.
Kiel University, Germany
Algorithmic Foundation of Genome Assembly
We give an introduction to genome assembly, a fundamental task in
biology and medicine. Using sequencing machines, huge amounts of data (TB or PB)
regarding the genome of one or more particular life forms are
gathered. This data must be evaluated, with the goal of determining the
correct genomic sequences present in the species under consideration.
The first giant sucess has been the assembly of the human genome
by C. Venter, M.D. Adams, E. W. Meyers et. al, Science 2001.
This work needed almost 10 years and supercomputing facilities.
Today, there is a demand for very fast assembly algorithms, even
for the size of the human genome, but running on smaller platforms, even on PCs.
We show how probabilistic data structures help to filter out redundant
information. Then, in the main part of the presentation, we turn to the
longest path problem. This is a classical problem in combinatorial
optimization and a prototype for algorithmic tasks that arise in genome
assembly. We discuss known approaches and then turn to the Big Data
aspect. An new algorithm for the longest path problem in the graph streaming
model is presented. We prove a linear per-edge processing time and show
experimental evidence that points towards a competitive solution quality
obtained within no more than 10 passes over the input, which is extremly small.
(joint work with Lasse Kliemann, Christian Schielke, and Axel Wedemeyer,
within the DFG SPP 1736 ,,Algorithms for Big Data´)
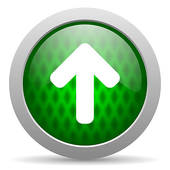
|
 | Manik Varma [Biography]
Manik Varma is a researcher at Microsoft Research India and an adjunct professor of computer science at the Indian Institute of Technology (IIT) Delhi. His research interests lie in the areas of machine learning, computational advertising and computer vision. He has served as an area chair for machine learning and computer vision conferences such as ACCV, CVPR, ICCV, ICML, ICVGIP, IJCAI and NIPS. Classifiers that he has designed are running live on millions of devices around the world protecting them from viruses and malware. He has been awarded the Microsoft Gold Star award, won the PASCAL VOC Object Detection Challenge and stood first in chicken chess tournaments and Pepsi drinking competitions. Manik is also a failed physicist (BSc St. Stephen's College, David Raja Ram Prize), theoretician (BA Oxford, Rhodes Scholar), engineer (DPhil Oxford, University Scholar) and mathematician (MSRI Berkeley, Post-doctoral Fellow).
Microsoft Research, India
Extreme Classification: A New Paradigm for Ranking & Recommendation
The objective in extreme multi-label classification is to learn a classifier that can automatically tag a data point with the most relevant subset of labels from a large label set. Extreme multi-label classification is an important research problem since not only does it enable the tackling of applications with many labels but it also allows the reformulation of ranking and recommendation problems with certain advantages over existing formulations.
Our objective, in this talk, is to develop an extreme multi-label classifier that is faster to train and more accurate at prediction than the state-of-the-art Multi-label Random Forest (MLRF) algorithm [Agrawal et al. WWW 13] and the Label Partitioning for Sub-linear Ranking (LPSR) algorithm [Weston et al. ICML 13]. MLRF and LPSR learn a hierarchy to deal with the large number of labels but optimize task independent measures, such as the Gini index or clustering error, in order to learn the hierarchy. Our proposed FastXML algorithm achieves significantly higher accuracies by directly optimizing an nDCG based ranking loss function. We also develop an alternating minimization algorithm for efficiently optimizing the proposed formulation. Experiments reveal that FastXML can be trained on problems with more than a million labels on a standard desktop in eight hours using a single core and in an hour using multiple cores.
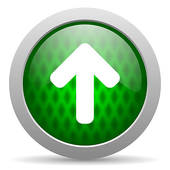
|
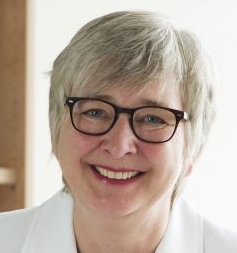 | Dorothea Wagner [Biography]
Dorothea Wagner heads the Institute of Theoretical Informatics at the Karlsruhe Institute of Technology. She earned her PhD 1986 from RWTH Aachen, and her habilitation 1992 from TU Berlin. Her research interests are in the field of graph algorithms and algorithm engineering with a focus on traffic optimization, social network analysis and network visualization. In 2012 she received the Google Focused Research Award for the project ""Next Generation Route Planner"". She is a member of several editoral boards and scientific advisory committees. From 2007 to 2014 she was vice president of the German Research Foundation (DFG). Since 2015 she is a member of the German Council of Science and Humanities.
Karlsruher Institut für Technologie, Germany
Route Planning for Time-Dependent Transportation
Nowadays, route planning systems belong to the most frequently used
information systems. The algorithmic core problem of such systems,
is the classical shortest paths problem that can be solved by Dijkstra's
algorithm which, however, is too slow for practical scenarios.
Algorithms for route planning in transportation networks have recently undergone a rapid development, leading to methods that are up to several million times faster than Dijkstra’s algorithm.
However, dealing with time-dependence is still a challenge.
This talk provides a condensed survey of recent advances in
algorithms for route planning in transportation networks. In
particular, we will discuss time-dependent scenarios including the process of building a time-dependent routing graph from raw road data like OpenStreetMap.
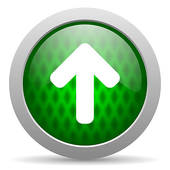
|
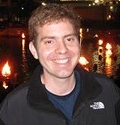 | David Woodruff [Biography]
David Woodruff joined IBM Almaden Research Center in 2007 right after completing his Ph.D. at MIT in theoretical computer science. He has been at IBM Almaden ever since. His research interests include communication complexity, data stream algorithms, machine learning, numerical linear algebra, sketching, and sparse recovery. He is the recipient of the 2014 Presburger Award and Best Paper Awards at STOC 2013 and PODS 2010. At IBM he is a member of the Academy of Technology and a Master Inventor.
IBM Almaden Research Center, USA
Sketching as a Tool for Linear Algebra and Recent Developments
We give near optimal algorithms for regression, low rank approximation, and robust variants of these problems. Our results are based on the sketch and solve paradigm, which is a tool for quickly compressing a problem to a smaller version of itself, for which one can then run a slow algorithm on the smaller problem. These lead to the fastest known algorithms for fundamental machine learning and numerical linear algebra problems, which run in time proportional to the number of non-zero entries of the input. We first give algorithms for least squares regression, and robust variants such as l_p regression and M-Estimator loss functions. Then we give algorithms for approximate singular value decomposition, and robust variants such as minimizing sum of distances to a subspace, rather than sum of squared distances. Time-permitting, we also discuss communication-efficient solutions to these problems in distributed environments.
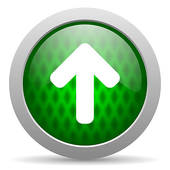
|
|